-
Notifications
You must be signed in to change notification settings - Fork 1.3k
Commit
This commit does not belong to any branch on this repository, and may belong to a fork outside of the repository.
- Loading branch information
Showing
3 changed files
with
160 additions
and
4 deletions.
There are no files selected for viewing
This file was deleted.
Oops, something went wrong.
This file contains bidirectional Unicode text that may be interpreted or compiled differently than what appears below. To review, open the file in an editor that reveals hidden Unicode characters.
Learn more about bidirectional Unicode characters
Original file line number | Diff line number | Diff line change |
---|---|---|
@@ -0,0 +1,159 @@ | ||
# 混合数据集训练 | ||
|
||
MMPose 提供了一个灵活、便捷的工具 `CombinedDataset` 来进行混合数据集训练。它作为一个封装器,可以包含多个子数据集,并将来自不同子数据集的数据转换成一个统一的格式,以用于模型训练。使用 `CombinedDataset` 的数据处理流程如下图所示。 | ||
|
||
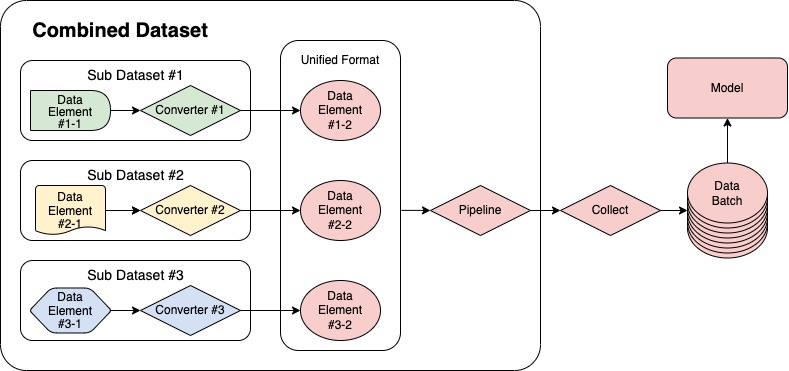 | ||
|
||
本篇教程的后续部分将通过一个结合 COCO 和 AI Challenger (AIC) 数据集的例子详细介绍如何配置 `CombinedDataset`。 | ||
|
||
## COCO & AIC 数据集混合案例 | ||
|
||
COCO 和 AIC 都是 2D 人体姿态数据集。但是,这两个数据集在关键点的数量和排列顺序上有所不同。下面是分别来自这两个数据集的图片及关键点: | ||
|
||
<img src="https://user-images.githubusercontent.com/26127467/223335806-748498af-8da4-4666-a6d3-337e4a8996f0.png" height="300px" alt><br> | ||
|
||
有些关键点(例如“左手”)在两个数据集中都有定义,但它们具有不同的序号。具体来说,“左手”关键点在 COCO 数据集中的序号为 9,在AIC数据集中的序号为 5。此外,每个数据集都包含独特的关键点,另一个数据集中不存在。例如,面部关键点(序号为0〜4)仅在 COCO 数据集中定义,而“头顶”(序号为 12)和“颈部”(序号为 13)关键点仅在 AIC 数据集中存在。以下的维恩图显示了两个数据集中关键点之间的关系。 | ||
|
||
<img src="https://user-images.githubusercontent.com/26127467/223338755-d838dd39-901b-4e7d-af8b-b94b5f5f9ef3.png" height="200px" alt><br> | ||
|
||
接下来,我们会介绍两种混合数据集的方式: | ||
|
||
- [将 AIC 合入 COCO 数据集](#将-aic-合入-coco-数据集) | ||
- [合并 AIC 和 COCO 数据集](#合并-aic-和-coco-数据集) | ||
|
||
### 将 AIC 合入 COCO 数据集 | ||
|
||
如果用户想提高其模型在 COCO 或类似数据集上的性能,可以将 AIC 数据集作为辅助数据。此时应该仅选择 AIC 数据集中与 COCO 数据集共享的关键点,忽略其余关键点。此外,还需要将这些被选择的关键点在 AIC 数据集中的序号进行转换,以匹配在 COCO 数据集中对应关键点的序号。 | ||
|
||
<img src="https://user-images.githubusercontent.com/26127467/223348541-d1f9e3b7-7e60-41b5-bf68-22e61b34bb2b.png" height="200px" alt><br> | ||
|
||
在这种情况下,来自 COCO 的数据不需要进行转换。此时 COCO 数据集可通过如下方式配置: | ||
|
||
```python | ||
dataset_coco = dict( | ||
type='COCODataset', | ||
data_root='data/coco/', | ||
ann_file='annotations/person_keypoints_train2017.json', | ||
data_prefix=dict(img='train2017/'), | ||
pipeline=[], # `pipeline` 应为空列表,因为 COCO 数据不需要转换 | ||
) | ||
``` | ||
|
||
对于 AIC 数据集,需要转换关键点的顺序。MMPose 提供了一个 `KeypointConverter` 转换器来实现这一点。以下是配置 AIC 子数据集的示例: | ||
|
||
```python | ||
dataset_aic = dict( | ||
type='AicDataset', | ||
data_root='data/aic/', | ||
ann_file='annotations/aic_train.json', | ||
data_prefix=dict(img='ai_challenger_keypoint_train_20170902/' | ||
'keypoint_train_images_20170902/'), | ||
pipeline=[ | ||
dict( | ||
type='KeypointConverter', | ||
num_keypoints=17, # 与 COCO 数据集关键点数一致 | ||
mapping=[ # 需要列出所有带转换关键点的序号 | ||
(0, 6), # 0 (AIC 中的序号) -> 6 (COCO 中的序号) | ||
(1, 8), | ||
(2, 10), | ||
(3, 5), | ||
(4, 7), | ||
(5, 9), | ||
(6, 12), | ||
(7, 14), | ||
(8, 16), | ||
(9, 11), | ||
(10, 13), | ||
(11, 15), | ||
]) | ||
], | ||
) | ||
``` | ||
|
||
`KeypointConverter` 会将原序号在 0 到 11 之间的关键点的序号转换为在 5 到 16 之间的对应序号。同时,在 AIC 中序号为为 12 和 13 的关键点将被删除。另外,目标序号在 0 到 4 之间的关键点在 `mapping` 参数中没有定义,这些点将被设为不可见,并且不会在训练中使用。 | ||
|
||
子数据集都完成配置后, 混合数据集 `CombinedDataset` 可以通过如下方式配置: | ||
|
||
```python | ||
dataset = dict( | ||
type='CombinedDataset', | ||
# 混合数据集关键点顺序和 COCO 数据集相同, | ||
# 所以使用 COCO 数据集的描述信息 | ||
metainfo=dict(from_file='configs/_base_/datasets/coco.py'), | ||
datasets=[dataset_coco, dataset_aic], | ||
# `train_pipeline` 包含了常用的数据预处理, | ||
# 比如图片读取、数据增广等 | ||
pipeline=train_pipeline, | ||
) | ||
``` | ||
|
||
MMPose 提供了一份完整的 [配置文件](https://github.com/open-mmlab/mmpose/blob/dev-1.x/configs/body_2d_keypoint/topdown_heatmap/coco/td-hm_hrnet-w32_8xb64-210e_coco-aic-256x192-merge.py) 来将 AIC 合入 COCO 数据集并用于训练网络。用户可以查阅这个文件以获取更多细节,或者参考这个文件来构建新的混合数据集。 | ||
|
||
### 合并 AIC 和 COCO 数据集 | ||
|
||
将 AIC 合入 COCO 数据集的过程中丢弃了部分 AIC 数据集中的标注信息。如果用户想要使用两个数据集中的所有信息,可以将两个数据集合并,即在两个数据集中取关键点的并集。 | ||
|
||
<img src="https://user-images.githubusercontent.com/26127467/223356617-075e0ab1-0ed3-426d-bc88-4f16be93f0ba.png" height="200px" alt><br> | ||
|
||
在这种情况下,COCO 和 AIC 数据集都需要使用 `KeypointConverter` 来调整它们关键点的顺序: | ||
|
||
```python | ||
dataset_coco = dict( | ||
type='COCODataset', | ||
data_root='data/coco/', | ||
ann_file='annotations/person_keypoints_train2017.json', | ||
data_prefix=dict(img='train2017/'), | ||
pipeline=[ | ||
dict( | ||
type='KeypointConverter', | ||
num_keypoints=19, # 并集中有 19 个关键点 | ||
mapping=[ | ||
(0, 0), | ||
(1, 1), | ||
# 省略 | ||
(16, 16), | ||
]) | ||
]) | ||
|
||
dataset_aic = dict( | ||
type='AicDataset', | ||
data_root='data/aic/', | ||
ann_file='annotations/aic_train.json', | ||
data_prefix=dict(img='ai_challenger_keypoint_train_20170902/' | ||
'keypoint_train_images_20170902/'), | ||
pipeline=[ | ||
dict( | ||
type='KeypointConverter', | ||
num_keypoints=19, # 并集中有 19 个关键点 | ||
mapping=[ | ||
(0, 6), | ||
# 省略 | ||
(12, 17), | ||
(13, 18), | ||
]) | ||
], | ||
) | ||
``` | ||
|
||
合并后的数据集有 19 个关键点,这与 COCO 或 AIC 数据集都不同,因此需要一个新的数据集描述信息文件。[coco_aic.py](https://github.com/open-mmlab/mmpose/blob/dev-1.x/configs/_base_/datasets/coco_aic.py) 是一个描述信息文件的示例,它基于 [coco.py](https://github.com/open-mmlab/mmpose/blob/dev-1.x/configs/_base_/datasets/coco.py) 并进行了以下几点修改: | ||
|
||
- 添加了 AIC 数据集的文章信息; | ||
- 在 `keypoint_info` 中添加了“头顶”和“颈部”这两个只在 AIC 中定义的关键点; | ||
- 在 `skeleton_info` 中添加了“头顶”和“颈部”间的连线; | ||
- 拓展 `joint_weights` 和 `sigmas` 以添加新增关键点的信息。 | ||
|
||
完成以上步骤后,合并数据集 `CombinedDataset` 可以通过以下方式配置: | ||
|
||
```python | ||
dataset = dict( | ||
type='CombinedDataset', | ||
# 使用新的描述信息文件 | ||
metainfo=dict(from_file='configs/_base_/datasets/coco_aic.py'), | ||
datasets=[dataset_coco, dataset_aic], | ||
# `train_pipeline` 包含了常用的数据预处理, | ||
# 比如图片读取、数据增广等 | ||
pipeline=train_pipeline, | ||
) | ||
``` | ||
|
||
此外,在使用混合数据集时,由于关键点数量的变化,模型的输出通道数也要做相应调整。如果用户用混合数据集训练了模型,但是要在 COCO 数据集上评估模型,就需要从模型输出的关键点中取出一个子集来匹配 COCO 中的关键点格式。可以通过 `test_cfg` 中的 `output_keypoint_indices` 参数自定义此子集。这个 [配置文件](https://github.com/open-mmlab/mmpose/blob/dev-1.x/configs/body_2d_keypoint/topdown_heatmap/coco/td-hm_hrnet-w32_8xb64-210e_coco-aic-256x192-combine.py) 展示了如何用 AIC 和 COCO 合并后的数据集训练模型并在 COCO 数据集上进行测试。用户可以查阅这个文件以获取更多细节,或者参考这个文件来构建新的混合数据集。 |
This file contains bidirectional Unicode text that may be interpreted or compiled differently than what appears below. To review, open the file in an editor that reveals hidden Unicode characters.
Learn more about bidirectional Unicode characters