forked from PaddlePaddle/Paddle
-
Notifications
You must be signed in to change notification settings - Fork 0
Commit
This commit does not belong to any branch on this repository, and may belong to a fork outside of the repository.
Add quant advanced tutorial (PaddlePaddle#634)
* add quant tutorial
- Loading branch information
Showing
5 changed files
with
217 additions
and
0 deletions.
There are no files selected for viewing
27 changes: 27 additions & 0 deletions
27
docs/zh_cn/tutorials/quant/paddleslim_quantization_overview.md
This file contains bidirectional Unicode text that may be interpreted or compiled differently than what appears below. To review, open the file in an editor that reveals hidden Unicode characters.
Learn more about bidirectional Unicode characters
Original file line number | Diff line number | Diff line change |
---|---|---|
@@ -0,0 +1,27 @@ | ||
# PaddleSlim模型量化方法总览 | ||
|
||
# 图像分类INT8量化模型在CPU上的部署和预测 | ||
PaddleSlim主要包含三种量化方法:量化训练(Quant Aware Training, QAT)、动态离线量化(Post Training Quantization Dynamic, PTQ Dynamic)、静态离线量化(Post Training Quantization Static, PTQ Static)。 | ||
|
||
- [量化训练](quant_aware_training_tutorial.md) 量化训练让模型感知量化运算对模型精度带来的影响,通过finetune训练降低量化误差。 | ||
- [动态离线量化](quant_post_dynamic_tutorial.md) 动态离线量化仅将模型中特定算子的权重从FP32类型映射成INT8/16类型。 | ||
- [静态离线量化](quant_post_static_tutorial.md) 静态离线量化使用少量无标签校准数据,采用KL散度等方法计算量化比例因子。 | ||
|
||
除此之外,PaddleSlim还有一种对embedding层量化的方法,将网络中embedding层参数从float32类型量化到int8类型。 | ||
|
||
- [Embedding量化](../static/embedding_quant_tutorial.md) Embedding量化仅将embedding参数从float32类型映射到int8类型,可以降低embedding参数体积。 | ||
|
||
下图展示了如何根据需要选择模型量化方法 | ||
|
||
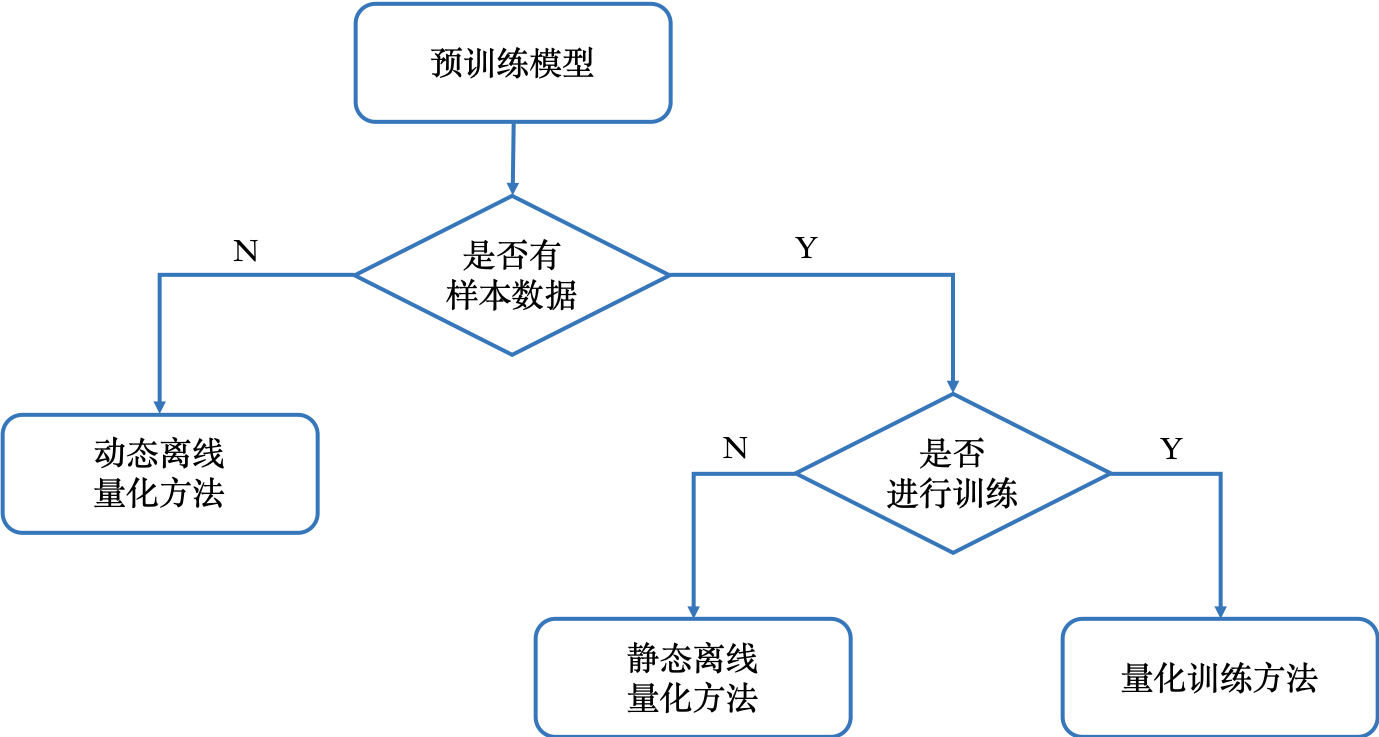 | ||
|
||
下表综合对比了模型量化方法的使用条件、易用性、精度损失和预期收益。 | ||
|
||
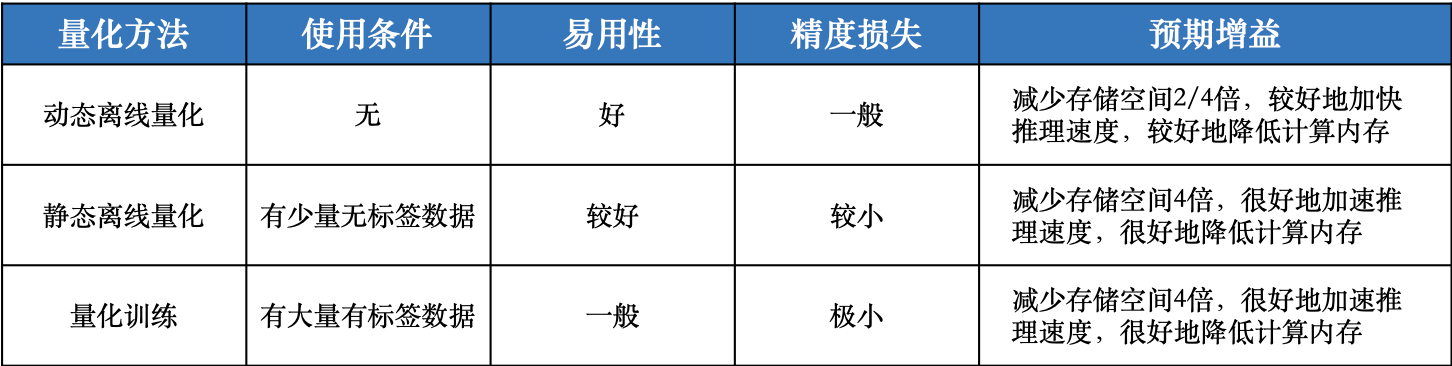 | ||
|
||
| 量化方法 | API接口 | 功能 | 经典适用场景 | | ||
| :------------------------------: | :----------------------------------------------------------: | :------------------------------------: | :---------------------------------------------: | | ||
| 在线量化 (QAT) | 动态图:`paddleslim.QAT`; 静态图:`paddleslim.quant.quant_aware` | 通过finetune训练将模型量化误差降到最小 | 对量化敏感的场景、模型,例如目标检测、分割, OCR | | ||
| 静态离线量化 (PTQ Static) | `paddleslim.quant.quant_post_static` | 通过少量校准数据得到量化模型 | 对量化不敏感的场景,例如图像分类任务 | | ||
| 动态离线量化 (PTQ Dynamic) | `paddleslim.quant.quant_post_dynamic` | 仅量化模型的可学习权重 | 模型体积大、访存开销大的模型,例如BERT模型 | | ||
| Embedding量化(Quant Embedding) | `paddleslim.quant.quant_embedding` | 仅量化模型的Embedding参数 | 任何包含Embedding层的模型 | |
106 changes: 106 additions & 0 deletions
106
docs/zh_cn/tutorials/quant/quant_aware_training_tutorial.md
This file contains bidirectional Unicode text that may be interpreted or compiled differently than what appears below. To review, open the file in an editor that reveals hidden Unicode characters.
Learn more about bidirectional Unicode characters
Original file line number | Diff line number | Diff line change |
---|---|---|
@@ -0,0 +1,106 @@ | ||
# 量化训练 | ||
|
||
在线量化是在模型训练的过程中建模定点量化对模型的影响,通过在模型计算图中插入量化节点,在训练建模量化对模型精度的影响降低量化损失。 | ||
|
||
|
||
PaddleSlim包含`QAT量化训练`和`PACT改进的量化训练`两种量化方法 | ||
|
||
- [QAT]() | ||
- [PACT]() | ||
|
||
## 使用方法 | ||
|
||
在线量化的基本流程可以分为以下四步: | ||
|
||
1. 选择量化配置 | ||
2. 转换量化模型 | ||
3. 启动量化训练 | ||
4. 保存量化模型 | ||
|
||
下面分别介绍以下几点: | ||
|
||
### 1. 选择量化配置 | ||
|
||
首先我们需要对本次量化的一些基本量化配置做一些选择,例如weight量化类型,activation量化类型等。如果没有特殊需求,可以直接拷贝我们默认的量化配置。全部可选的配置可以参考PaddleSlim量化文档,例如我们用的量化配置如下: | ||
|
||
```python | ||
quant_config = { | ||
'weight_preprocess_type': None, | ||
'activation_preprocess_type': None, | ||
'weight_quantize_type': 'channel_wise_abs_max', | ||
'activation_quantize_type': 'moving_average_abs_max', | ||
'weight_bits': 8, | ||
'activation_bits': 8, | ||
'dtype': 'int8', | ||
'window_size': 10000, | ||
'moving_rate': 0.9, | ||
'quantizable_layer_type': ['Conv2D', 'Linear'], | ||
} | ||
``` | ||
|
||
### 2. 转换量化模型 | ||
|
||
在确认好我们的量化配置以后,我们可以根据这个配置把我们定义好的一个普通模型转换为一个模拟量化模型。我们根据量化原理中介绍的PACT方法,定义好PACT函数pact和其对应的优化器pact_opt。在这之后就可以进行转换,转换的方式也很简单: | ||
|
||
```python | ||
import paddleslim | ||
quanter = paddleslim.QAT(config=quant_config) | ||
quanter.quantize(net) | ||
``` | ||
|
||
### 3. 启动量化训练 | ||
|
||
得到了量化模型后就可以启动量化训练了,量化训练与普通的浮点数模型训练并无区别,无需增加新的代码或逻辑,直接按照浮点数模型训练的流程进行即可。 | ||
|
||
### 4. 保存量化模型 | ||
|
||
量化训练结束后,我们需要对量化模型做一个转化。PaddleSlim会对底层的一些量化OP顺序做调整,以便预测使用。转换及保存的基本流程如下所示: | ||
|
||
```python | ||
import paddleslim | ||
quanter.save_quantized_model( | ||
model, | ||
path, | ||
input_spec=[paddle.static.InputSpec()]) | ||
``` | ||
|
||
## PACT在线量化 | ||
|
||
PACT方法是对普通在线量化方法的改进,对于一些量化敏感的模型,例如MobileNetV3,PACT方法一般都能降低量化模型的精度损失。 | ||
|
||
使用方法上与普通在线量化方法相近: | ||
|
||
```python | ||
# 在quant_config中额外指定'weight_preprocess_type'为'PACT' | ||
quant_config = { | ||
'weight_preprocess_type': 'PACT', | ||
'weight_quantize_type': 'channel_wise_abs_max', | ||
'activation_quantize_type': 'moving_average_abs_max', | ||
'weight_bits': 8, | ||
'activation_bits': 8, | ||
'dtype': 'int8', | ||
'window_size': 10000, | ||
'moving_rate': 0.9, | ||
'quantizable_layer_type': ['Conv2D', 'Linear'], | ||
} | ||
|
||
``` | ||
|
||
详细代码与例程请参考: | ||
|
||
- [动态图量化训练](https://github.com/PaddlePaddle/PaddleSlim/tree/develop/demo/dygraph/quant) | ||
|
||
## 实验结果 | ||
|
||
| 模型 | 压缩方法 | 原模型Top-1/Top-5 Acc | 量化模型Top-1/Top-5 Acc | | ||
| :---------------: | :--------------: | :-------------------: | :---------------------: | | ||
| MobileNetV1 | quant_aware | 70.99%/89.65% | 70.63%/89.65% | | ||
| MobileNetV2 | quant_aware | 72.15%/90.65% | 72.05%/90.63% | | ||
| ResNet50 | quant_aware | 76.50%/93.00% | 76.48%/93.11% | | ||
| MobileNetV3_large | pact_quant_aware | 78.96%/94.48% | 77.52%/93.77% | | ||
|
||
## 参考文献 | ||
|
||
1. [Quantizing deep convolutional networks for efficient inference: A whitepaper](https://arxiv.org/pdf/1806.08342.pdf) | ||
|
||
2. [PACT: Parameterized Clipping Activation for Quantized Neural Networks](https://arxiv.org/abs/1805.06085) |
This file contains bidirectional Unicode text that may be interpreted or compiled differently than what appears below. To review, open the file in an editor that reveals hidden Unicode characters.
Learn more about bidirectional Unicode characters
Original file line number | Diff line number | Diff line change |
---|---|---|
@@ -0,0 +1,27 @@ | ||
# 动态离线量化 | ||
|
||
动态离线量化,将模型中特定OP的权重从FP32类型量化成INT8/16类型。 | ||
|
||
量化前需要有训练好的预测模型,可以根据需要将模型转化为INT8或INT16类型,目前只支持反量化预测方式,主要可以减小模型大小,对特定加载权重费时的模型可以起到一定加速效果。 | ||
|
||
- 权重量化成INT16类型,模型精度不受影响,模型大小为原始的1/2。 | ||
- 权重量化成INT8类型,模型精度会受到影响,模型大小为原始的1/4。 | ||
|
||
## 使用方法 | ||
|
||
- 准备预测模型:先保存好FP32的预测模型,用于量化压缩。 | ||
- 产出量化模型:使用PaddlePaddle调用动态离线量化离线量化接口,产出量化模型。 | ||
|
||
主要代码实现如下: | ||
|
||
```python | ||
import paddleslim | ||
model_dir = path/to/fp32_model_params | ||
save_model_dir = path/to/save_model_path | ||
paddleslim.quant.quant_post_dynamic(model_dir=model_dir, | ||
save_model_dir=save_model_dir, | ||
weight_bits=8, | ||
quantizable_op_type=['conv2d', 'mul'], | ||
weight_quantize_type="channel_wise_abs_max", | ||
generate_test_model=False) | ||
``` |
This file contains bidirectional Unicode text that may be interpreted or compiled differently than what appears below. To review, open the file in an editor that reveals hidden Unicode characters.
Learn more about bidirectional Unicode characters
Original file line number | Diff line number | Diff line change |
---|---|---|
@@ -0,0 +1,40 @@ | ||
# 静态离线量化 | ||
|
||
静态离线量化是基于采样数据,采用KL散度等方法计算量化比例因子的方法。相比量化训练,静态离线量化不需要重新训练,可以快速得到量化模型。 | ||
|
||
静态离线量化的目标是求取量化比例因子,主要有两种方法:非饱和量化方法 ( No Saturation) 和饱和量化方法 (Saturation)。非饱和量化方法计算FP32类型Tensor中绝对值的最大值`abs_max`,将其映射为127,则量化比例因子等于`abs_max/127`。饱和量化方法使用KL散度计算一个合适的阈值`T` (`0<T<mab_max`),将其映射为127,则量化比例因子等于`T/127`。一般而言,对于待量化op的权重Tensor,采用非饱和量化方法,对于待量化op的激活Tensor(包括输入和输出),采用饱和量化方法 。 | ||
|
||
## 使用方法 | ||
|
||
静态离线量化的实现步骤如下: | ||
|
||
- 加载预训练的FP32模型,配置reader; | ||
- 读取样本数据,执行模型的前向推理,保存待量化op激活Tensor的数值; | ||
- 基于激活Tensor的采样数据,使用饱和量化方法计算它的量化比例因子; | ||
- 模型权重Tensor数据一直保持不变,使用非饱和方法计算它每个通道的绝对值最大值,作为每个通道的量化比例因子; | ||
- 将FP32模型转成INT8模型,进行保存。 | ||
|
||
主要代码实现如下: | ||
|
||
```python | ||
import paddleslim | ||
exe = paddle.static.Executor(place) | ||
paddleslim.quant.quant_post( | ||
executor=exe, | ||
model_dir=model_path, | ||
quantize_model_path=save_path, | ||
sample_generator=reader, | ||
model_filename=model_filename, | ||
params_filename=params_filename, | ||
batch_nums=batch_num) | ||
``` | ||
|
||
详细代码与例程请参考:[静态离线量化](https://github.com/PaddlePaddle/PaddleSlim/tree/develop/demo/quant/quant_post) | ||
|
||
## 实验结果 | ||
|
||
| 模型 | 压缩方法 | 原模型Top-1/Top-5 Acc | 量化模型Top-1/Top-5 Acc | | ||
| :---------------: | :--------------: | :-------------------: | :---------------------: | | ||
| MobileNetV1 | quant_post_static | 70.99%/89.65% | 70.18%/89.25% | | ||
| MobileNetV2 | quant_post_static | 72.15%/90.65% | 71.15%/90.11% | | ||
| ResNet50 | quant_post_static | 76.50%/93.00% | 76.33%/93.02% | |
This file contains bidirectional Unicode text that may be interpreted or compiled differently than what appears below. To review, open the file in an editor that reveals hidden Unicode characters.
Learn more about bidirectional Unicode characters
Original file line number | Diff line number | Diff line change |
---|---|---|
@@ -0,0 +1,17 @@ | ||
# Embedding量化 | ||
|
||
Embedding量化将网络中的Embedding参数从`float32`类型量化到 `8-bit`整数类型,在几乎不损失模型精度的情况下减少模型的存储空间和显存占用。 | ||
|
||
Embedding量化仅能减少模型参数的体积,并不能显著提升模型预测速度。 | ||
## 使用方法 | ||
|
||
在预测时调用paddleslim `quant_embedding`接口,主要实现代码如下: | ||
|
||
```python | ||
import paddleslim | ||
place = paddle.CUDAPlace(0) if use_cuda else paddle.CPUPlace() | ||
exe = paddle.static.Executor(place) | ||
main_program = paddleslim.quant.quant_embedding(main_program, place, config) | ||
``` | ||
|
||
详细代码与例程请参考:[Embedding量化](https://github.com/PaddlePaddle/PaddleSlim/tree/develop/demo/quant/quant_embedding) |