-
Notifications
You must be signed in to change notification settings - Fork 272
New issue
Have a question about this project? Sign up for a free GitHub account to open an issue and contact its maintainers and the community.
By clicking “Sign up for GitHub”, you agree to our terms of service and privacy statement. We’ll occasionally send you account related emails.
Already on GitHub? Sign in to your account
signal extractor SlidingWindowMaxSum #1568
Merged
kosack
merged 15 commits into
cta-observatory:master
from
jsitarek:implement_SlidingWindowMaxSum
Feb 11, 2021
Merged
Changes from 6 commits
Commits
Show all changes
15 commits
Select commit
Hold shift + click to select a range
beab6d5
a new signal extractor, slightly slower, but with better accuracy (in…
jsitarek 4adb6ae
added missing apply_integration_correction variable to SlidingWindowM…
jsitarek 3cc405f
adding a standard test of the newly added single extractor SlidingWin…
jsitarek 1849d7d
added the test for the extract_sliding_window function that is used b…
jsitarek 7e2844a
fixing a few small issues from codacy scan
jsitarek c9c327e
fixing two trailing whitespaces
jsitarek 439c203
follow up commit on PR #1568
jsitarek 093dca3
Merge branch 'master' of https://github.com/cta-observatory/ctapipe i…
jsitarek d3528e6
moved the dirty fix of the LST pulse shape to monkeypatch
jsitarek 8a2bce9
solving codacy warnings in PR 1588
jsitarek 84effe4
resolving codacy issues
jsitarek 69749bf
Merge remote-tracking branch 'upstream/master' into implement_Sliding…
jsitarek bf2d2e4
follow up of PR 1568
jsitarek ae70502
fixed an error left in the previous commit that would fail the test
jsitarek 7798779
corrected indentation
jsitarek File filter
Filter by extension
Conversations
Failed to load comments.
Loading
Jump to
Jump to file
Failed to load files.
Loading
Diff view
Diff view
There are no files selected for viewing
This file contains bidirectional Unicode text that may be interpreted or compiled differently than what appears below. To review, open the file in an editor that reveals hidden Unicode characters.
Learn more about bidirectional Unicode characters
This file contains bidirectional Unicode text that may be interpreted or compiled differently than what appears below. To review, open the file in an editor that reveals hidden Unicode characters.
Learn more about bidirectional Unicode characters
Add this suggestion to a batch that can be applied as a single commit.
This suggestion is invalid because no changes were made to the code.
Suggestions cannot be applied while the pull request is closed.
Suggestions cannot be applied while viewing a subset of changes.
Only one suggestion per line can be applied in a batch.
Add this suggestion to a batch that can be applied as a single commit.
Applying suggestions on deleted lines is not supported.
You must change the existing code in this line in order to create a valid suggestion.
Outdated suggestions cannot be applied.
This suggestion has been applied or marked resolved.
Suggestions cannot be applied from pending reviews.
Suggestions cannot be applied on multi-line comments.
Suggestions cannot be applied while the pull request is queued to merge.
Suggestion cannot be applied right now. Please check back later.
There was a problem hiding this comment.
Choose a reason for hiding this comment
The reason will be displayed to describe this comment to others. Learn more.
Could you implement this directly here, does not sound to complicated?
There was a problem hiding this comment.
Choose a reason for hiding this comment
The reason will be displayed to describe this comment to others. Learn more.
the main reason why I did not do so is because this feature does not seem to be used (at least in LST), so I did not have a proper set-up to test it, but I can look into making some dummy pulse shape and testing on it.
There was a problem hiding this comment.
Choose a reason for hiding this comment
The reason will be displayed to describe this comment to others. Learn more.
that would be great
There was a problem hiding this comment.
Choose a reason for hiding this comment
The reason will be displayed to describe this comment to others. Learn more.
You have the reference pulse shape in the
CameraDescription
. I guess it's a fairly small effect though, and the correction doesn't really matter much except to get the cleaning thresholds in the same units for all cameras.There was a problem hiding this comment.
Choose a reason for hiding this comment
The reason will be displayed to describe this comment to others. Learn more.
Sorry for the delay, I restarted working on this.
CameraDescription.readout is where the code is taking the pulse shape from. However this is not really reliable.
If I execute the following code
I get the following figure:
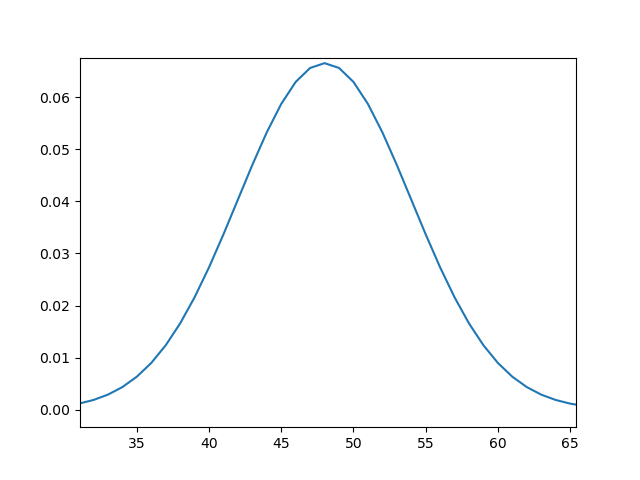
which is a much broader pulse then it should
The calculation of the correction factor would be much simpler if the pulse shape in this class had the same binning as the actual readout, this is the case in the above example, and one would assume to take it from granted since the shape is taken from the "readout" object, which has the binning embedded, however in the first tests that i was doing in lstchain, when the array was being read from the data the pulse shapes there were actually a delta function with a SSC-like sampling, so obviously it cannot be taken for granted.
I will change the code to use a simple conversion and rounding of sampling to make it work also in this more general case, but the whole issue of the LST pulse shape deserves a separate "issue"
EDIT: I forgot to mention that there seems to be only one reference pulse shape in the CameraDescription, while in reality we should have HG and LG
There was a problem hiding this comment.
Choose a reason for hiding this comment
The reason will be displayed to describe this comment to others. Learn more.
The
from_name()
methods load up a file from ctapipe-extra (which is now a directory on a server rather than a package), and are just meant for unit-testing purposes. Currently everything in ctapipe-extra is from PROD3 or even PROD2 simulations, so quite out of date for real analysis. In the future I want to clean that up and have an option to select which "prod" to use, but there has not been manpower for that (see e.g #738 )If you load real data from a SimTel file or something else supported, the correct waveform should be loaded into the instrument model that you get from
source.subarray
.E.g. if you do:
You will get the "latest" pulse that is defined in Prod5
There was a problem hiding this comment.
Choose a reason for hiding this comment
The reason will be displayed to describe this comment to others. Learn more.
Note that if you don't want to always load up a file, you can take any reference prod5 file, for example, and run
And it will dump a bunch of FITS files including the Camera geometry and readout definitions to the local directory. You can then setenv
CTAPIPE_SVC_PATH=[directory where those files are]
, and ctapipe will use that when you run thefrom_name()
functions instead of the defaults (by default it searches all paths listed in a ":" separated list in in CTAPIPE_SVC_PATH first, then if it doesn't find that, it will download the default file from the dataserver, which as I said are a bit out of date.There was a problem hiding this comment.
Choose a reason for hiding this comment
The reason will be displayed to describe this comment to others. Learn more.
Update: I found the actual problem... In fact, the camera readout definition for LSTCam does not even exist in the ctapipe-extra directory on the dataserver. It seems the default behavior is to just return some dummy pulse shape if the file is not found, which has no real meaning (you should see a logger warning message if logging is set up)... Clearly this is not good behavior (I think it was there to prevent tests from failing until we updated the testing files, which never happened).
here is a comparison with prod3b:
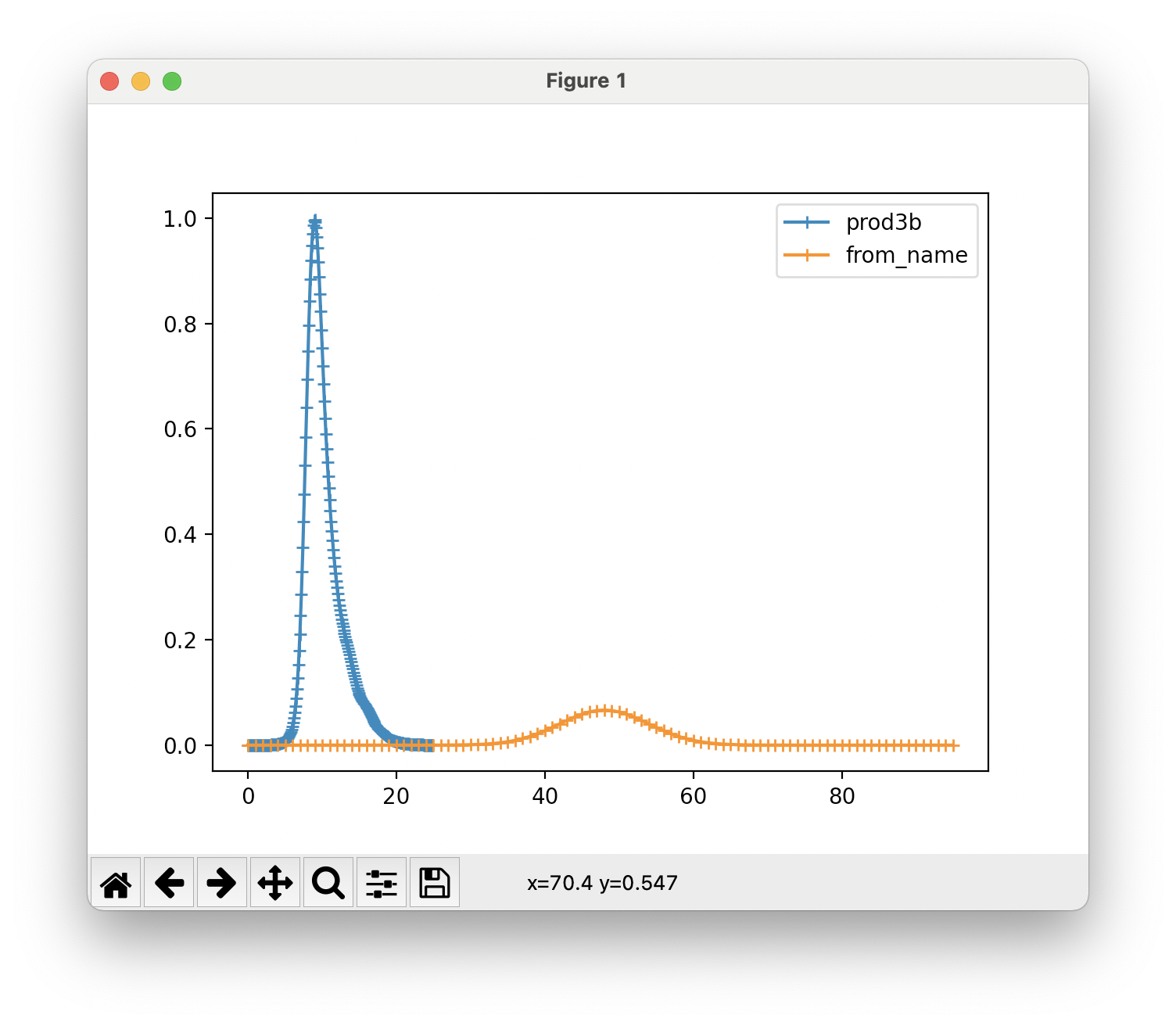
There was a problem hiding this comment.
Choose a reason for hiding this comment
The reason will be displayed to describe this comment to others. Learn more.
This is also why you get only 1 reference pulse shape if you use
from_name()
...I had already opened an issue about this, but obviously forgot: See #1450