-
Notifications
You must be signed in to change notification settings - Fork 73
New issue
Have a question about this project? Sign up for a free GitHub account to open an issue and contact its maintainers and the community.
By clicking “Sign up for GitHub”, you agree to our terms of service and privacy statement. We’ll occasionally send you account related emails.
Already on GitHub? Sign in to your account
eva函数存在问题 #17
Comments
感谢你指出我们代码里的问题。 首先说这个评价函数我也是用的别人的,但是我确实忘了源代码是从哪里看的了。我用的时候也没注意到这个问题,并不是我们为了效果好而刻意这么做的。 其次我们论文里所有的实验都是在y_pred和y_true是相同类别数的条件下做的实验,所以函数中 后续我会再确定一下之前的代码来源,然后修改源文件中的代码。再次感谢你的提醒。 |
感谢你的回复,指出这个问题的本意也是为了让学习者们可以更好的学习和玩这个模型。
我相信这应该不是你们的本意,但是难免会存在一些特例,使得模型的y_pred与y_true不相同,我在别的issue里也看到有eva函数报错的情况,其本质问题就是来源于这里。
最开始我在想为什么不直接使用sklearn里的acc计算函数accuracy_score,后来我发现accuracy_score有一个致命的问题就是无法去映射类别,比如1 1 2 2 和2 2 1 1 的序列,在聚类上其实是可以认为是分类对的,但是accuracy_score由于其通用性,会认为这是错误的。
你这边使用的这个函数正是为了解决这类问题而存在的,通过映射的方式去改变序列使得accuracy_score可以得到预期的结果,但可能编写者并不严谨,从而存在一些问题,比如在类别无法完全匹配的情况下,使用不恰当的方式去强行匹配。
我尝试改写了下代码,通过在后面追加缺失类别的label值来保障其后面使用匈牙利算法时无法匹配上的问题,使得他可以正常work的同时,不会出现上述的问题,源码附在邮件中,当然这也是一种取巧的方式,且并没有很严谨的证明其完全没问题,依然有可能存在一些没考虑到的情况,但不失为可以参考的做法。当然也很期待你能修改出或找到更好的解决方式。
最后十分感谢你百忙之中关注到这个问题并给予回复,也衷心祝福你在学术上能继续有所进展,希望可以继续看到你的文章,从中看到更有趣的世界。机器学习是一件有趣的事情,很高兴能和你交谈。
…------------------ 原始邮件 ------------------
发件人: "bdy9527/SDCN" ***@***.***>;
发送时间: 2021年4月1日(星期四) 下午3:56
***@***.***>;
***@***.******@***.***>;
主题: Re: [bdy9527/SDCN] eva函数存在问题 (#17)
感谢你指出我们代码里的问题。
首先说这个评价函数我也是用的别人的,但是我确实忘了源代码是从哪里看的了。我用的时候也没注意到这个问题,并不是我们为了效果好而刻意这么做的。
其次我们论文里所有的实验都是在y_pred和y_true是相同类别数的条件下做的实验,所以函数中if numclass1 != numclass2这个判断是不会触发的,也就是说我们并没有在评测过程中修改pred来得到更好的结果。
后续我会再确定一下之前的代码来源,然后修改源文件中的代码。再次感谢你的提醒。
—
You are receiving this because you authored the thread.
Reply to this email directly, view it on GitHub, or unsubscribe.
|
|
@thirteenbird 您好,感谢您的细致分析,看了您的分析并使用了您的代码。可是当我在实际使用时, 通过代码计算的acc与通过混淆矩阵计算的acc会降低一点都,F1 rel与pre 指标则相差很多,当用混淆矩阵手动计算时,这三类指标都比通过此代码计算高很多,当使用macro参数时,F1 rec与pre 指标则特别低,rec相差近特别特别多,我更换了几次标签实验发现还是如此,您可以给我一些帮助吗? 简单来说,acc会降低3个百分点,F1 rec与pre 则相差很多。检查了输入的两列标签与对照变换后的标签并没有发现问题,十分感谢您。 |
@mant-ux 我个人猜测,造成这个的原因可能是我这种逃课的方式,在y_true和pred类别对不上的时候,匈牙利算法做映射时因为增加了新的行导致映射的不对,从而存在这种问题
如果y_true和pred类别数量一直的话,那应该不会存在问题?因为相当于使用匈牙利算法映射后,用sklearn.metrics库做的统计指标计算,应该会和人工使用混淆矩阵一致 |
@thirteenbird 感谢您的回复,我检查了标签并没有问题,并且通过代码映射变换后的标签与真实标签做混淆矩阵类别数也是一致的,但是代码得到的rec只有8%,混淆矩阵计算得到的大道了70%,acc相差代码低了3%,是我标签处理的问题吗? |
在evaluation.py文件里面的评价函数eva,调用了自己写的acc/f1函数,里面存在很大的问题
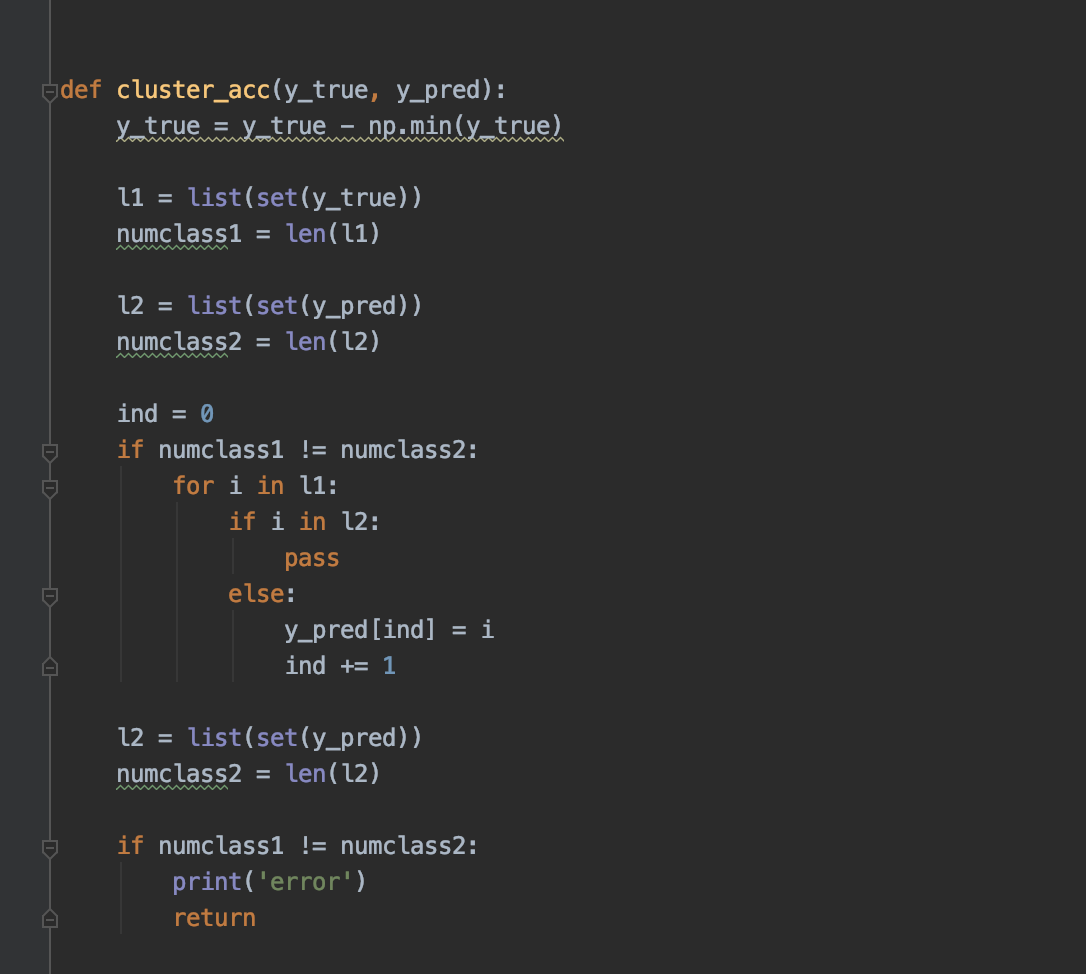
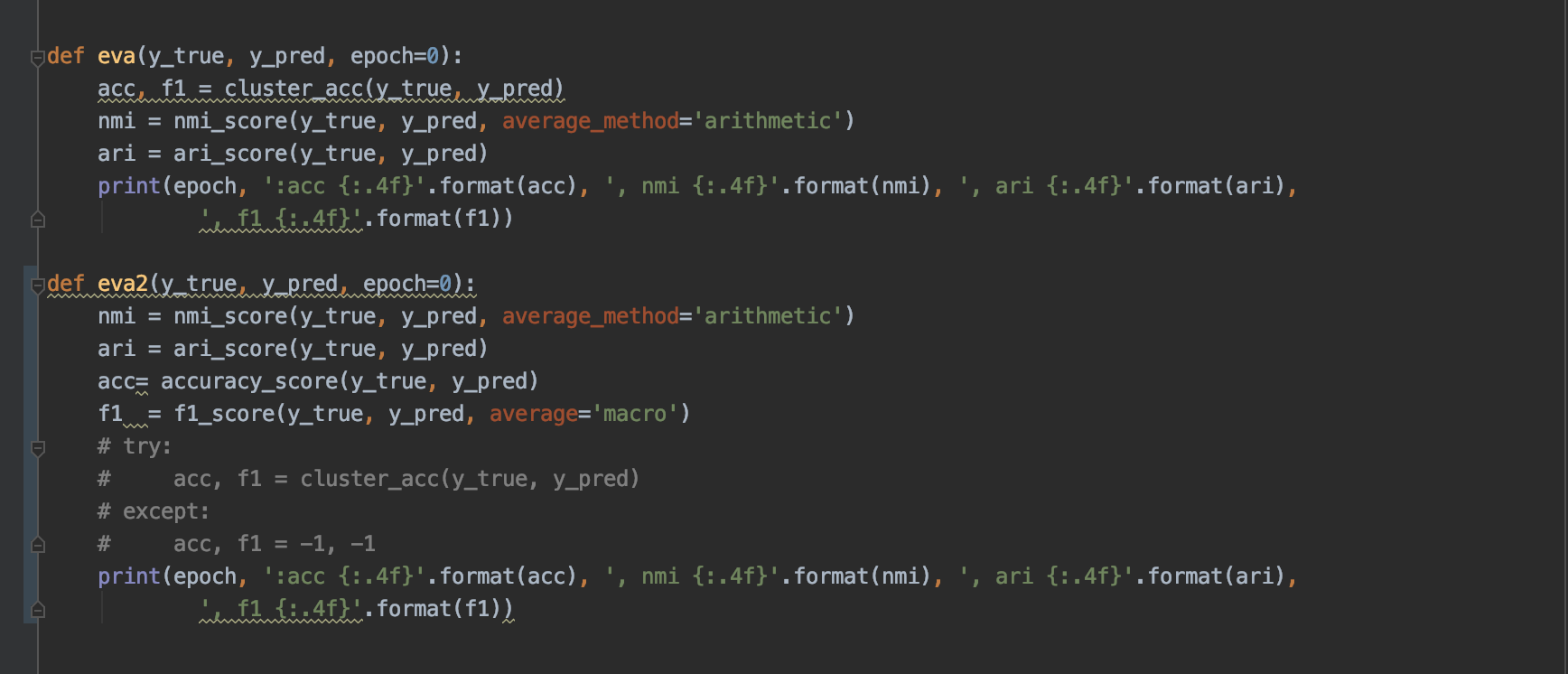
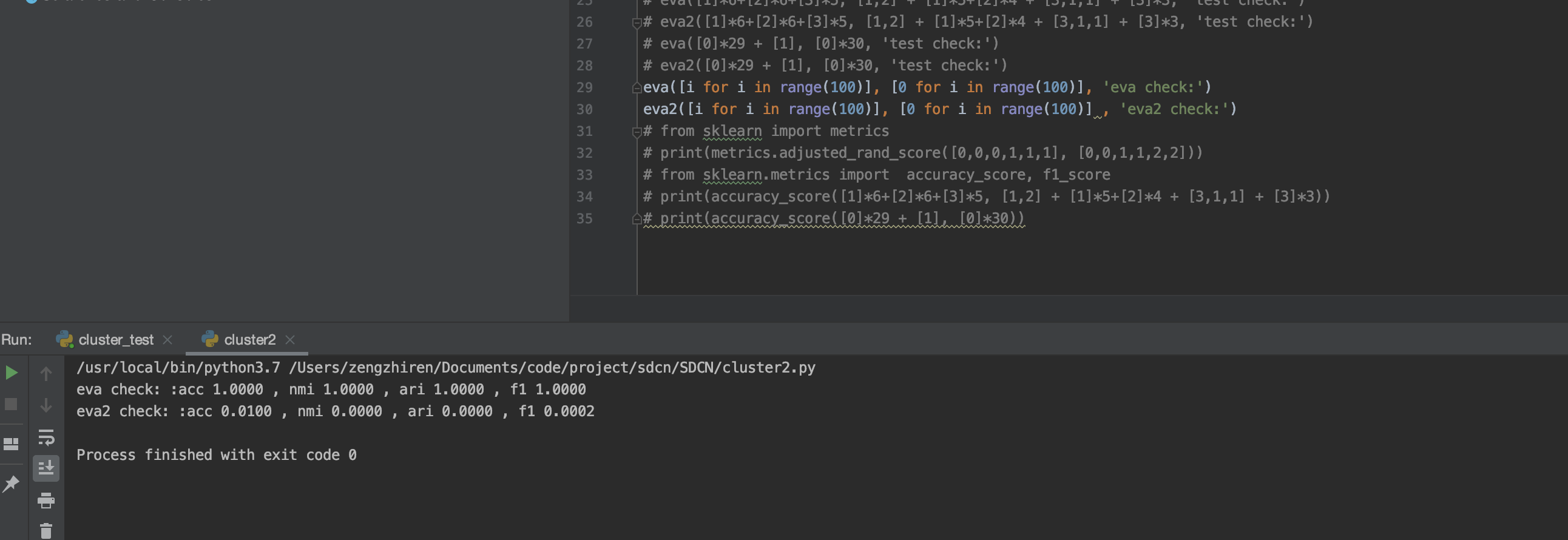
代码中的acc函数如下
在两者类不匹配的时候,会去改写pred的结果,而且这是直接修改原始数据,会导致后续的nmi/ari计算使用被修改了的pred数据,使得acc/nmi/ari/f1这四个评估指标与真实存在差异
比如如下,使用sklearn.metrics提供的指标计算函数
在自行构造的特殊场景下,有着很大的差别,假设存在100个点,并且类别都不一样,其原始分类为[0, ..., 100],现在假设全都预测为同一类,此时正常的eval应该是很差的,但是evaluation.py里的eva函数的数据结果却为极好
The text was updated successfully, but these errors were encountered: