You signed in with another tab or window. Reload to refresh your session.You signed out in another tab or window. Reload to refresh your session.You switched accounts on another tab or window. Reload to refresh your session.Dismiss alert
Hi Pytorch team, recently I need to calculate per sample's gradient with respect to part of model's parameters. The problem is that for the toy example, it works. But for the Wide & Deep model, it doesn't work and returns me all 0 gradients. I don't know why.
Also, in the Wide & Deep module, the shape of linear_logit should be (batch_size,1), but when apply this method, the error will happened here, and the system said the shape of linear_logit and sparse_feat_logit is not match, here I attached the print out result. (I suppose when using this method, the X.shape[0]=0, but why?)
Hi Pytorch team, recently I need to calculate per sample's gradient with respect to part of model's parameters. The problem is that for the toy example, it works. But for the Wide & Deep model, it doesn't work and returns me all 0 gradients. I don't know why.
Here is the toy example:
The result is :
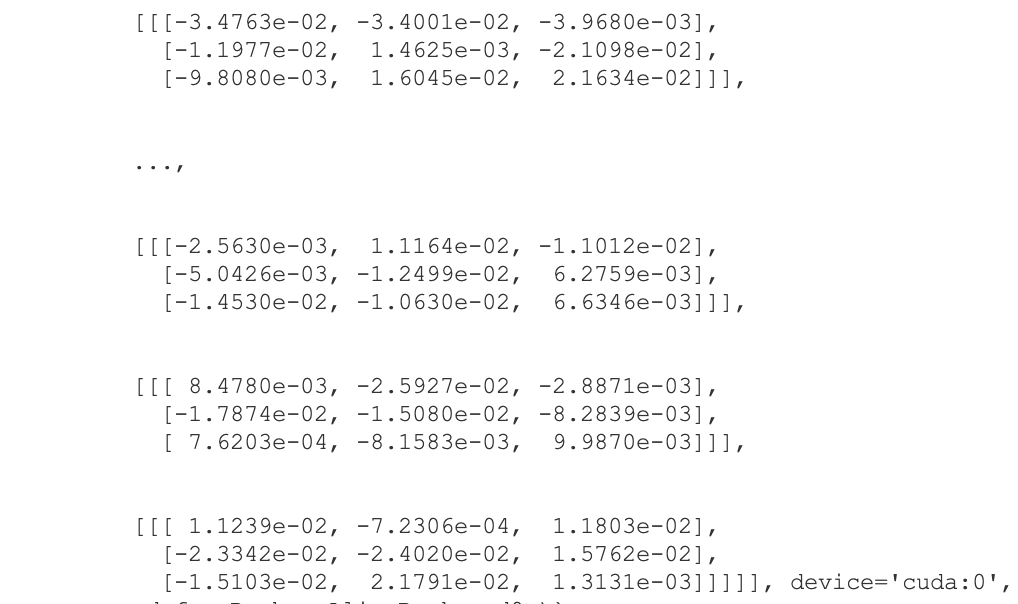
However, when I apply this method to the real scenario, it doesn't works and all return 0 gradient.
Also, in the Wide & Deep module, the shape of linear_logit should be (batch_size,1), but when apply this method, the error will happened here, and the system said the shape of linear_logit and sparse_feat_logit is not match, here I attached the print out result. (I suppose when using this method, the X.shape[0]=0, but why?)
The text was updated successfully, but these errors were encountered: