-
-
Notifications
You must be signed in to change notification settings - Fork 0
/
slides_1.html
524 lines (406 loc) · 21.1 KB
/
slides_1.html
1
2
3
4
5
6
7
8
9
10
11
12
13
14
15
16
17
18
19
20
21
22
23
24
25
26
27
28
29
30
31
32
33
34
35
36
37
38
39
40
41
42
43
44
45
46
47
48
49
50
51
52
53
54
55
56
57
58
59
60
61
62
63
64
65
66
67
68
69
70
71
72
73
74
75
76
77
78
79
80
81
82
83
84
85
86
87
88
89
90
91
92
93
94
95
96
97
98
99
100
101
102
103
104
105
106
107
108
109
110
111
112
113
114
115
116
117
118
119
120
121
122
123
124
125
126
127
128
129
130
131
132
133
134
135
136
137
138
139
140
141
142
143
144
145
146
147
148
149
150
151
152
153
154
155
156
157
158
159
160
161
162
163
164
165
166
167
168
169
170
171
172
173
174
175
176
177
178
179
180
181
182
183
184
185
186
187
188
189
190
191
192
193
194
195
196
197
198
199
200
201
202
203
204
205
206
207
208
209
210
211
212
213
214
215
216
217
218
219
220
221
222
223
224
225
226
227
228
229
230
231
232
233
234
235
236
237
238
239
240
241
242
243
244
245
246
247
248
249
250
251
252
253
254
255
256
257
258
259
260
261
262
263
264
265
266
267
268
269
270
271
272
273
274
275
276
277
278
279
280
281
282
283
284
285
286
287
288
289
290
291
292
293
294
295
296
297
298
299
300
301
302
303
304
305
306
307
308
309
310
311
312
313
314
315
316
317
318
319
320
321
322
323
324
325
326
327
328
329
330
331
332
333
334
335
336
337
338
339
340
341
342
343
344
345
346
347
348
349
350
351
352
353
354
355
356
357
358
359
360
361
362
363
364
365
366
367
368
369
370
371
372
373
374
375
376
377
378
379
380
381
382
383
384
385
386
387
388
389
390
391
392
393
394
395
396
397
398
399
400
401
402
403
404
405
406
407
408
409
410
411
412
413
414
415
416
417
418
419
420
421
422
423
424
425
426
427
428
429
430
431
432
433
434
435
436
437
438
439
440
441
442
443
444
445
446
447
448
449
450
451
452
453
454
455
456
457
458
459
460
461
462
463
464
465
466
467
468
469
470
471
472
473
474
475
476
477
478
479
480
481
482
483
484
485
486
487
488
489
490
491
492
493
494
495
496
497
498
499
500
501
502
503
504
505
506
507
508
509
510
511
512
513
514
515
516
517
518
519
520
521
522
523
524
<!doctype html>
<html lang="en">
<head>
<meta charset="utf-8">
<title>Lecture 1 - NNML 2020</title>
<meta name="description" content="NNML">
<meta name="author" content="Emre Neftci">
<meta name="apple-mobile-web-app-capable" content="yes">
<meta name="apple-mobile-web-app-status-bar-style" content="black-translucent">
<meta name="viewport" content="width=device-width, initial-scale=1.0">
<link rel="stylesheet" href="dist/reset.css">
<link rel="stylesheet" href="dist/reveal.css">
<link rel="stylesheet" href="dist/theme/nmilab.css" id="theme">
<!-- Theme used for syntax highlighting of code -->
<link rel="stylesheet" href="plugin/highlight/monokai.css" id="highlight-theme">
<link rel="stylesheet" type="text/css" href="https://cdn.rawgit.com/dreampulse/computer-modern-web-font/master/fonts.css">
<link rel="stylesheet" type="text/css" href="https://maxcdn.bootstrapcdn.com/font-awesome/4.5.0/css/font-awesome.min.css">
<link rel="stylesheet" type="text/css" href="lib/css/monokai.css">
<link
rel="stylesheet"
href="https://cdnjs.cloudflare.com/ajax/libs/animate.css/4.0.0/animate.min.css"
/>
<link rel="stylesheet" href="reveal.js-plugins/appearance/appearance.css" />
<link rel="stylesheet" href="plugin/highlight/monokai.css" id="highlight-theme" />
<!-- Printing and PDF exports -->
<script>
var link = document.createElement( 'link' );
link.rel = 'stylesheet';
link.type = 'text/css';
link.href = window.location.search.match( /print-pdf/gi ) ? 'css/print/pdf.css' : 'css/print/paper.css';
document.getElementsByTagName( 'head' )[0].appendChild( link );
</script>
<script async defer src="https://buttons.github.io/buttons.js"></script>
</head>
<body>
<div class="reveal">
<!-- Any section element inside of this container is displayed as a slide -->
<div class="slides">
<section data-external="title.html" data-vertical-align-top data-background-color=#B2BA67 >
</section>
<section data-markdown data-vertical-align-top><textarea data-template>
## Example: Keyword Detection


- Every second, the microphone will collect roughly 44,000 samples. Each sample is a measurement of the amplitude of the sound wave
- How to detect "Alexa" , "OK, Google" from the raw audio?
</textarea>
</section>
<section data-markdown data-vertical-align-top><textarea data-template>
## Example: Face Detection


- Every image is a collection of numbers indicating the intensity of the color channel at a given pixel
- How to detect the presence of a face in a picture?
</textarea>
</section>
<section data-markdown data-vertical-align-top><textarea data-template>
## Machine Learning Approach

<ul>
<li /> The goal of Machine Learning (ML) is to learn to solve a problem by extracting patterns from raw data
<li class=fragment /> The performance of ML models depends heavily on the representation of the data
<li class=fragment /> Neural Networks are particularly efficient at learning these representations
</ul>
</textarea></section>
<section data-markdown data-vertical-align-top><textarea data-template>
## History of Artificial Intelligence and Neural Networks

<div class=fragment>
<p>Early AI shortcomings: </p>
<ul>
<li/> Symbol based processing lacks domain-specific knowledge
<li/> Combinatorial explosion: solutions to small problems did not scale to exponentially large problems.
<li/> Solving a problem in principle is very different than solving it practically
</ul>
</div>
</textarea>
</section>
<section data-markdown><textarea data-template>
## AI's Moonshot
<img src=images/image.I6QZC0.png class="stretch"/>
</textarea>
</section>
<section>
<h2> Modern Artificial Intelligence and Machine Learning </h2>
<img src=images/image.X8Q2C0.png />
<img src=images/image.0IJ5C0.png />
<div class="fragment fade-in"><p class="pl">A lot of progress in machine learning can be attributed to better hardware and
more data</p></div>
</section>
<section>
<h2>Connectionism and Neural Networks</h2><ul>
<img src="images/connectionnism.png"/>
<li/>At the heart of deep learning, there is an <b>artificial neural network</b></li>
<li/>Artificial neural networks are a subset of machine learning approaches using networks of simple (neuron-like) units.</li>
</ul>
</section>
<section data-markdown><textarea data-template>
## Machine Learning / Artificial Intelligence
<img src="images/venn_ml.png" class=stretch />
<blockquote>Deep learning is a kind of representation learning, which is in turn a kind of machine learning, which is used for many but not all approaches to AI" </blockquote>
<p class='ref'>(Goodfellow et al. 2016)</p>
</textarea>
</section>
<section data-markdown><textarea data-template>
## How does deep learning differ from other AI systems?
<img src=images/ml_flowchart.png class="stretch"/>
<p class='ref'>(Goodfellow et al. 2016)</p>
</textarea>
</section>
<section data-markdown><textarea data-template>
## Learning from Examples
- Machine learning typically uses (large) ***datasets*** to learn to
- Recognize patterns (Classification)
- Generate patterns (Generation)
- Take Actions (Reinforcement Learning)
</textarea>
</section>
<section data-markdown><textarea data-template>
## Visual Recognition
- Visual Recognition (Image Classification) is the most common task performed by neural networks

- Neural networks are trained using a dataset consisting of pairs of images and labels
</textarea>
</section>
<section data-markdown><textarea data-template>
## Example Dataset: MNIST and CIFAR
<div class="row">
<div class="column">
<p style="text-align:center" >MNIST</p>
<img src=images/mnist.png />
</div>
<div class="column">
<p style="text-align:center" >CIFAR10</p>
<img src=images/CIFAR-10.png />
</div>
</div>
- MNIST/CIFAR10 are considered as the "Hello, World" example of deep learning
</textarea>
</section>
<section data-markdown><textarea data-template>
## Example Dataset: ImageNet

- ImageNet: 1M images, 1000 classes, 469x387 pixels
<img src=images/ilsvrc.png class=small />
[](http://www.image-net.org/)
</textarea></section>
<section data-markdown><textarea data-template>
## Example Dataset: CelebA

- CelebA: large-scale face attributes dataset with more than 200K celebrity images, each with 40 attribute annotations.
</textarea>
</section>
<section data-markdown><textarea data-template>
## Beyond Image Classification
Machine Learning and neural networks can be applied to a wide variety of problems, such as:
- Image Segmentation and Object Detection
- Text or Audio Classification
- Regression
- Translation
- Anomaly Detection
- Generation (Density Estimation)
</textarea></section>
<section data-markdown><textarea data-template>
## Other (Non-Visual) Datasets:
- Speech Commands: 65,000 one-second long utterances of 30 short words, such as "Yes", "No", "Right", "Stop"
- Penn Tree Bank (PTB) dataset: A text corpus that is parsed and annotated for natural language processing research <!-- .element: class="fragment" -->
- SMS Spam Collection Dataset: 5574 messages, tagged according being ham (legitimate) or spam <!-- .element: class="fragment" -->
</textarea>
</section>
<section data-markdown><textarea data-template>
## Convolutional Neural Networks: Image Classification
<img src="images/image_classification_imagenet.png" class="small"/>
<img src="images/lenet.png" class=stretch />
</textarea></section>
<section data-markdown><textarea data-template>
## YOLO: Object Detection
<img src="images/yolo.png" class="stretch"/>
</textarea></section>
<section data-markdown><textarea data-template>
## U-Nets: Image Segmentation
<img src="images/u-net-segmentation.png" class="small"/>
<img src="images/u-net-architecture.png" class="stretch" />
</textarea></section>
<section data-markdown><textarea data-template>
## Neural Style Transfer
<img src=images/art_style_transfer.png class=stretch />
</textarea></section>
<section data-markdown><textarea data-template>
## Neural Style Transfer: Deep Empathy
<img src=images/deep_empathy_style_transfer.png class="stretch" />
</textarea></section>
<section data-markdown><textarea data-template>
## Image Generation Using Generative Adversarial Networks (GAN)
<img src=images/image_generation_gan.png class=stretch />
</textarea></section>
<section data-markdown><textarea data-template>
## Visual Attention: Captioning
<img src=images/captioning.png class=stretch />
</textarea></section>
<section data-markdown><textarea data-template>
## Deep Reinforcement Learning: Game of Go
<img src=images/game_playing.png class=stretch />
<p class=ref>Silver, et al. 2016</p>
</textarea></section>
<section data-markdown><textarea data-template>
## Sequence Prediction
- Data can have a temporal structure
- Neural networks can be applied to learn and predict sequences
- Recurrent Neural Networks are one such example
<img src=images/sequence_prediction.png />
<p class=ref>C. Olah, 2015</p>
</textarea></section>
<section data-markdown><textarea data-template>
## Robot Control: Solving Rubik's Cube with a Robot Hand
<div class=row>
<div class=column>
<img src="images/openai_rubiks_arch.png" style="height:600px;max-height:600px" />
</div>
<div class=column>
<iframe src="https://www.youtube.com/embed/kVmp0uGtShk" frameborder="0" allow="accelerometer; autoplay; encrypted-media; gyroscope; picture-in-picture" allowfullscreen style='autoplay; max-height:500px;height:300px;width:100%;translate: scale(.1)'></iframe>
<p class=ref>OpenAI, 2019</p>
</div>
</div>
</textarea></section>
<section data-markdown><textarea data-template>
## WaveNets: Voice and Music Generation
<img src=images/wavenet.png class=stretch />
- Parametric Text-To-Speech <audio controls="" src="https://storage.googleapis.com/deepmind-media/research/WaveNet/US%20English/parametric-1.wav"></audio>
- WaveNet <audio controls="" src="https://storage.googleapis.com/deepmind-media/research/WaveNet/US%20English/wavenet-1.wav"></audio>
<p class=ref>Van den Oord et al. 2016</p>
</textarea></section>
<section data-markdown><textarea data-template>
## Attention Networks: Machine Translation
<img src=images/translation.png />
<img src="images/attention_bahdenau.png" class=stretch />
<p class=ref>Bahdenau, et al. 2015</p>
</textarea></section>
<section data-markdown><textarea data-template>
## ML/NN as Models for Understanding the Brain
<img src="images/comparing_ann_and_brain.png" class=stretch />
<p class=ref>Blake, et al. 2019</p>
- ML/NN attempts to solve tasks that are similar to that of animals.
- Researchers use ML/NN to make hypotheses in the brain.
</textarea></section>
<section data-markdown><textarea data-template>
## The Explosion of Deep Learning
- Deep Learning has become the de facto solution for any representation learning problem
- Neural Information Processing Systems (NeurIPS) is the most prestigious conference in ML/AI
- 13'000 Participant in 2019
- In 2018, the main conference sold out after 12 minutes
<div class="row">
<div class="column">
<img src=images/neurips_stats.png />
</div>
<div class="column">
<img src=images/neurips2019_photo.JPG />
</div>
</div>
</textarea>
</section>
<section data-markdown><textarea data-template>
## Books
<ul>
<li /> Machine Learning
<ul>
<li/> Ian Goodfellow, Yoshua Bengio, and Aaron Courville. Deep learning. MIT press, 2016.
<li/> C.M. Bishop. Pattern recognition and machine learning. Springer-Verlag New York, Inc. Secaucus, NJ, USA, 2006.
</ul>
<li class=fragment /> Biologically-Inspired Neural Networks
<ul>
<li/> Wulfram Gerstner, Werner M Kistler, Richard Naud, and Liam Paninski. Neuronal dynamics: From single neurons to networks and models of cognition. Cambridge University Press, 4.
<li/> E. O. Neftci, H. Mostafa, and F. Zenke. “Surrogate Gradient Learning in Spiking Neural Networks: Bringing the Power of Gradient-Based Optimization to Spiking Neural Networks”. IEEE Signal Processing Magazine 36.6 (Nov. 2019), pp. 51–63.
</ul>
</textarea>
</section>
<section data-markdown><textarea data-template>
## Course Overview (Weeks 1-3)
- Historical perspective and Course logistics (Today)
- Pattern Recognition Basics (1 Week) <!-- .element: class="fragment" -->
- Linear Regression, Classification (k-Nearest Neighbor, Perceptrons, Multilayer Perceptrons)
- Machine Learning and Deep Learning (2 Weeks) <!-- .element: class="fragment" -->
- Software and computer setup
- Deep Neural Networks
- Loss functions
- Optimization, Gradient Backpropagation
</textarea>
</section>
<section data-markdown><textarea data-template>
## Course Overview (Weeks 5-10)
- Applications (4 weeks)
- Visual Recognition: ConvNets
- Pattern Generation: Autoencoders, Variational Autoencoders and Generative Adversarial Networks
- Sequence Learning: Recurrent Neural Networks, LSTM, WaveNet
- Natural Language Processing: Embeddings, Transformer Networks
- From Artificial Neural Networks to Biological Neural Nets: (1 week) <!-- .element: class="fragment" -->
- Spiking Neural Networks
- Surrogate Gradient Learning
- Final Projects Presentation (Last week) <!-- .element: class="fragment" -->
</textarea>
</section>
<section data-markdown><textarea data-template>
## Software and Assignments
This is a hands-on class comprising programming assignments. All programming will be browser-based (no installation necessary):
- Programming Language: Python 3
[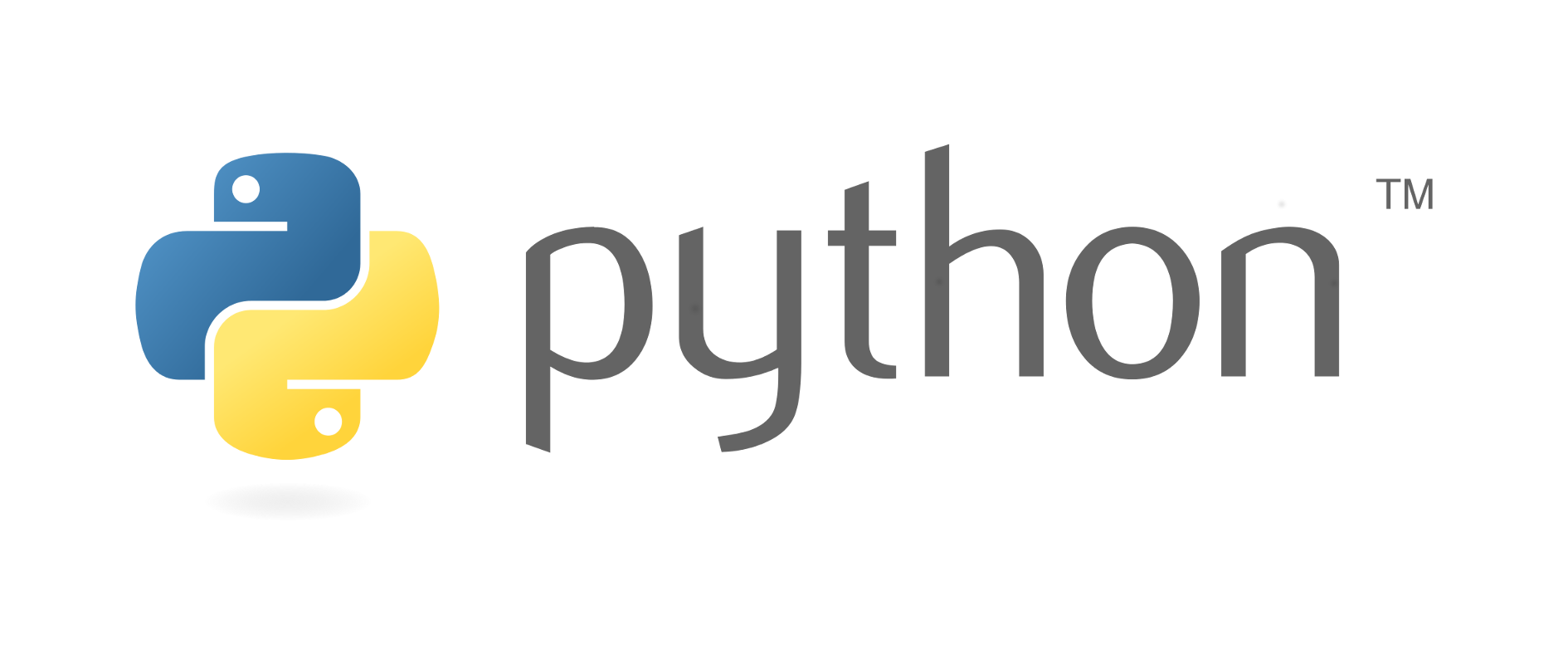](https://scipy-lectures.org/intro/)
- Expected basic scientific programming skills in Python, and familiarity with the concepts in sections 1.1 through 1.4 in the [scipy lecture notes](http://www.scipy-lectures.org/intro/index.html).
- Programming Environment: Jupyter + Google Colaboratory
[](https://colab.research.google.com/notebooks/welcome.ipynb)
- Deep Learning Environment: PyTorch
[](http://pytorch.org)
</textarea>
</section>
<section data-markdown><textarea data-template>
## Final Project
- Topic in ML/NN:
- Apply a neural network to a new dataset, or
- Modify an algorithm on an existing dataset
- Week 10: 10+5 min presentation
- Finals Week: Project report, using NeurIPS conference paper template https://nips.cc/Conferences/2015/PaperInformation/StyleFiles
- Discuss topic with instructor before week 6
- Groups of two OK, but delineate contributions
</textarea>
</section>
<section data-markdown><textarea data-template>
## Final Project: Example Projects
- Visual / Sequence Recognition
- Terrain Classification from Videos
- Agent Localization using Video
- Computational Neuroscience
- Representation Similarity Analysis with Binary Neural Networks
- Classification/Regression on neuroimaging data
- Artifact detection using EEG
- Representation Similarity Analysis using Convnets and behavioral and neuroimaging data
<p class="pl">If possible, choose a topic that relates to your expertise and research</p>
</textarea>
</section>
</section>
</div>
</div>
<script src="dist/reveal.js"></script>
<script src="plugin/zoom/zoom.js"></script>
<script src="plugin/notes/notes.js"></script>
<script src="plugin/math/math.js"></script>
<script src="plugin/search/search.js"></script>
<script src="plugin/markdown/markdown.js"></script>
<script src="plugin/highlight/highlight.js"></script>
<script src="reveal.js-plugins/menu/menu.js"></script>
<script src="reveal.js-plugins/audio-slideshow/plugin.js"></script>
<script src="reveal.js-plugins/audio-slideshow/recorder.js"></script>
<script src="reveal.js-plugins/audio-slideshow/RecordRTC.js"></script>
<script src="reveal.js-plugins/chalkboard/plugin.js"></script>
<script src="reveal.js-plugins/external/external.js"></script>
<script src="reveal.js-plugins/externalcode/externalcode.js"></script>
<script src="reveal.js-plugins/appearance/appearance.js"></script>
<script>
Reveal.configure({ pdfMaxPagesPerSlide: 1, hash: true, slideNumber: true})
// Also available as an ES module, see:
// https://revealjs.com/initialization/
Reveal.initialize({
mouseWheel: false,
width: 1280,
height: 720,
margin: 0.0,
navigationMode: 'grid',
transition: 'fade',
controls: true,
progress: true,
center: true,
hash: true,
// Learn about plugins: https://revealjs.com/plugins/
plugins: [Appearance, RevealZoom, RevealSearch, RevealMarkdown, RevealHighlight, RevealAudioRecorder, RevealAudioSlideshow, RevealMath, RevealExternal, Externalcode, RevealMenu],
menu: { // Menu works best with font-awesome installed: sudo apt-get install fonts-font-awesome
themes: false,
transitions: false,
markers: true,
hideMissingTitles: true,
custom: [
{ title: 'Plugins', icon: '<i class="fa fa-external-link-alt"></i>', src: 'toc.html' },
{ title: 'About', icon: '<i class="fa fa-info"></i>', src: 'about.html' }
]
},
audio: {
prefix: 'audio/slides_1/', // audio files are stored in the "audio" folder
suffix: '.ogg', // audio files have the ".ogg" ending
textToSpeechURL: null, // the URL to the text to speech converter
defaultNotes: false, // use slide notes as default for the text to speech converter
defaultText: false, // use slide text as default for the text to speech converter
advance: 300, // advance to next slide after given time in milliseconds after audio has played, use negative value to not advance
autoplay: false, // automatically start slideshow
defaultDuration: 1.0, // default duration in seconds if no audio is available
defaultAudios: true, // try to play audios with names such as audio/1.2.ogg
playerOpacity: 0.5, // opacity value of audio player if unfocused
playerStyle: 'position: fixed; bottom: 4px; left: 25%; width: 50%; height:75px; z-index: 33;', // style used for container of audio controls
startAtFragment: false, // when moving to a slide, start at the current fragment or at the start of the slide
},
// ...
keyboard: {
82: function() { Recorder.toggleRecording(); }, // press 'r' to start/stop recording
90: function() { Recorder.downloadZip(); }, // press 'z' to download zip containing audio files
84: function() { Recorder.fetchTTS(); } // press 't' to fetch TTS audio files
}
});
</script>
</body>
</html>