-
Notifications
You must be signed in to change notification settings - Fork 36
New issue
Have a question about this project? Sign up for a free GitHub account to open an issue and contact its maintainers and the community.
By clicking “Sign up for GitHub”, you agree to our terms of service and privacy statement. We’ll occasionally send you account related emails.
Already on GitHub? Sign in to your account
Improving performance for h5netcdf #195
Comments
As an example, the following patch, avoids the creation of the diff --git a/h5netcdf/core.py b/h5netcdf/core.py
index 39b0b5a..cb7f2b1 100644
--- a/h5netcdf/core.py
+++ b/h5netcdf/core.py
@@ -114,6 +114,10 @@ class BaseVariable(object):
self._dimensions = dimensions
self._initialized = True
+ # Always refer to the root file and store not h5py object
+ # subclasses:
+ self._h5ds = self._root._h5file[self._h5path]
+
@property
def _parent(self):
return self._parent_ref()
@@ -122,12 +126,6 @@ class BaseVariable(object):
def _root(self):
return self._root_ref()
- @property
- def _h5ds(self):
- # Always refer to the root file and store not h5py object
- # subclasses:
- return self._root._h5file[self._h5path]
-
@property
def name(self):
"""Return variable name."""
diff --git a/h5netcdf/dimensions.py b/h5netcdf/dimensions.py
index d291731..d5bda4d 100644
--- a/h5netcdf/dimensions.py
+++ b/h5netcdf/dimensions.py
@@ -86,8 +86,13 @@ class Dimension(object):
else:
self._root._max_dim_id += 1
self._dimensionid = self._root._max_dim_id
- if parent._root._writable and create_h5ds and not self._phony:
+ if self._phony:
+ self._h5ds = None
+ elif parent._root._writable and create_h5ds:
+ # Create scale will create the self._h5ds object
self._create_scale()
+ else:
+ self._h5ds = self._root._h5file[self._h5path]
self._initialized = True
@property
@@ -131,12 +136,6 @@ class Dimension(object):
return False
return self._h5ds.maxshape == (None,)
- @property
- def _h5ds(self):
- if self._phony:
- return None
- return self._root._h5file[self._h5path]
-
@property
def _isscale(self):
return h5py.h5ds.is_scale(self._h5ds.id)
@@ -183,6 +182,7 @@ class Dimension(object):
dtype=">f4",
**kwargs,
)
+ self._h5ds = self._root._h5file[self._h5path]
self._h5ds.attrs["_Netcdf4Dimid"] = np.array(self._dimid, dtype=np.int32)
if len(self._h5ds.shape) > 1:
|
Relevant discussion: #117 |
@hmaarrfk Thanks for providing all that information and your engagement to make h5netcdf more performant. I've taken a first step to minimize re-reading from underlying h5py/h5df in #196. The changes are minor, but have a huge effect on the presented use-case. I've tried your patch here, but it broke the test suite. I'll look deeper into this the next days. |
Thank you! My patch was more a proof of concept that I also think that we (personally) need to tune our file structure. I remember reading about HDF5 metadata needing to be tuned for "large" files. Were you able to recreate similar performance trends with a newly created hand crafted file? We likely generated the original netcdf4 with |
@hmaarrfk Do you think it would be useful to create several datasets from scratch with netCDF4-python/h5netcdf? We could build a benchmark suite with your and others specific use cases from that, also including examples from the past. At least this would give us some comparison between different approaches. |
Building benchmarking infrastructure is non-trivial. I'm happy to help creating the datasets, and "donating" some "real world" dataset. |
For this particular case, I found that it isn't dependent on my own nc file import h5netcdf
from pathlib import Path
from time import perf_counter, sleep
import tempfile
with tempfile.TemporaryDirectory() as tmpdir:
filename = Path(tmpdir) / 'h5netcdf_test.nc'
h5nc_file = h5netcdf.File(filename, 'w')
h5nc_file.dimensions = {'x': 1024}
x = h5nc_file.dimensions['x']
time_start = perf_counter()
for i in range(1000):
len(x)
time_end = perf_counter()
time_elapsed = time_end - time_start
print(f"{time_elapsed:03.3f} --- Time to compute length 1000 times: ")
time_start = perf_counter()
for i in range(1000):
x._h5ds
time_end = perf_counter()
time_elapsed = time_end - time_start
print(f"{time_elapsed:03.3f} --- Time to access h5ds reference 1000 times")
time_start = perf_counter()
for i in range(1000):
x._root._h5file[x._h5path]
time_end = perf_counter()
time_elapsed = time_end - time_start
print(f"{time_elapsed:03.3f} --- Time to create h5ds reference 1000 times")
h5nc_file.close()
# %% on main
# 0.053 --- Time to compute length 1000 times:
# 0.046 --- Time to access h5ds reference 1000 times
# 0.046 --- Time to create h5ds reference 1000 times
# %% On https://github.com/h5netcdf/h5netcdf/pull/197
# 0.003 --- Time to compute length 1000 times:
# 0.000 --- Time to access h5ds reference 1000 times
# 0.034 --- Time to create h5ds reference 1000 times |
I think I might be encountering the same issue when using xarray with def name(self):
""" Return the full name of this object. None if anonymous. """
return self._d(h5i.get_name(self.id)) However, the netcdf dataset I am testing with has >2410 variables and the call to |
I'm not sure if i cross referenced it here, but ultimately some of my speedups came in conflict with some file caching I couldn't figure out. I tried to document it pydata/xarray#7359 |
@hmaarrfk it seems that your xarray question is not being answered and the PR from @kmuehlbauer would be a useful fix but was also not merged. What is the best way to go forward? Is it realistic that either of these will be picked up further on the short term? |
I'm honestly not sure. I've tried to understand the xarray caching file manager. It doesn't seem super intuitive to edit. I feel like for many of my use cases, we've someewhat learned to live with it. Sometimes it helps to learn that other people care. I open alot of issues on OSS projects, so sometimes its easy to think that "Mark is just opening them for awareness". For example, I still owe this metadata: |
I encourage you to comment on the issues I linked to. |
@hmaarrfk I cannot judge the last issue you linked, as for much of the other linked PR's and issues. I would gladly comment on issues if that seems helpful (I did on the one you linked just yet), but not sure where and who. I hope the open PR's/issues can be consolidated a bit and that the separate efforts can be combined. |
What happened:
I've been trying to use h5netcdf since it gives access to the underlying
h5py
dataset which makes it easier to prototype with different HDF5 backends. netcdf4-c is much harder to compile and thus prototype with since many of the internals are not accessible.My original concern arose from observations that, for some of our datasets, they open with
Ultimately, I believe that I think things boil down to the fact an access to the
_h5ds
property is quite costly. This makes "every" operation "slow".the code below:
Using the spyder profile, one finds that 1000 calls to size, result in about 8000 calls to
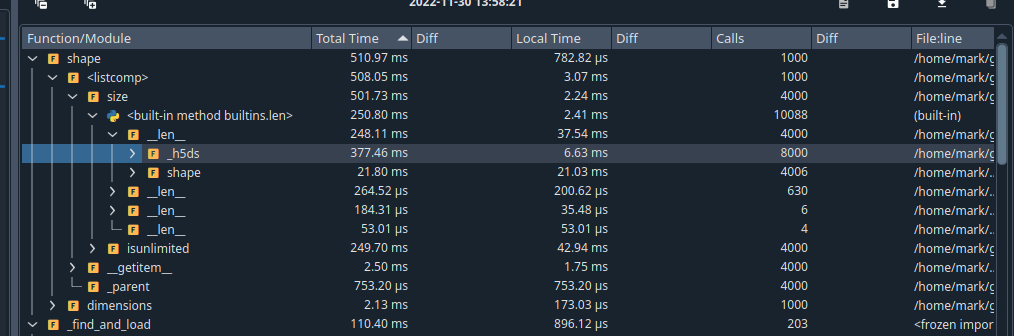
_h5ds
This is because this property is created on demand.
I think this has cascading effects because everything really relies on the access to the underlying
h5py
structure.A previous suggestion tried to remove the property, but it seems like there was some concern about "resetting" the underlying
_h5py
pointer. I am unclear on where this kind of usecase would come up. My understanding is that this pointer would be something unchanging.Expected Output
As a comparison, we can compare how long it takes to access the shape property from netcdf4:
About 400 us instead of 350 ms.
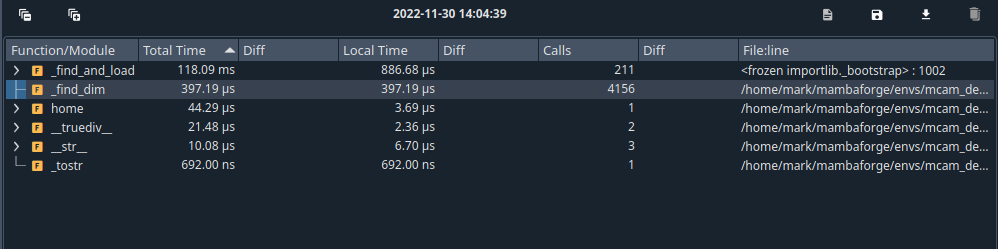
Anything else we need to know?:
The sample image has 4 dimensions
sample_file_zebrafish_0.18.55_4uCams_small.zip
Version
Output of print(h5py.version.info, f"\nh5netcdf {h5netcdf.__version__}")
Summary of the h5py configuration
h5py 3.7.0
HDF5 1.12.2
Python 3.9.13 | packaged by ...
[GCC 10.4.0]
sys.platform linux
sys.maxsize 9223372036854775807
numpy 1.23.5
cython (built with) 0.29.32
numpy (built against) 1.20.3
HDF5 (built against) 1.12.2
h5netcdf 0.14.0.dev51+g8302776
The text was updated successfully, but these errors were encountered: