You signed in with another tab or window. Reload to refresh your session.You signed out in another tab or window. Reload to refresh your session.You switched accounts on another tab or window. Reload to refresh your session.Dismiss alert
I am trying to train the coarsenet to see if it can learn to produce images from very sparse point clouds. Here is my series of steps:
I load the pretrained coarsent model ('wts/depth_sift_rgb/coarsenet.model.npz') and a suitable annotations file (containing very few examples).
Providing a low percentage of points to be used (between 5 and 10), I let it run for some iterations (around 10000) without changing any other default hyper-parameters.
To check the results, I run the demo_5k.ply, after changing the coarsenet model to one obtained from above. First, I run into the following error, which I think is because the saved weights do not have the mean and variances (for eg 'ec0_mn', 'ec0_vr') saved for each layer, as provided in the pre-trained weights. If anyone has successfully directly used modified weights, it wold be really helpful.
I try to circumvent the above error by explicitly loading the corsenet model with batchnorm set to 'set' and then doing the following:
C.load(sess, cnet_input)
for k in C.bn_outs:
C.weights[k] = C.bn_outs[k]
Running with these weights gives bogus results (uniformly grey images as shown below). I wonder how the weights have shifted so much in just a few iterations starting from pre-trained weights which give great results.
I tried with the example annotation file as well and without the sparsity constraints but it led to similar results. So basically, if I sart from pretrained weights, the model seems to just degrade very quickly even using the same training data. think I might be doing something wrong as these results are strange. Has anyone been successful in training over additional data starting from pre-trained weights ?
The text was updated successfully, but these errors were encountered:
I am trying to train the coarsenet to see if it can learn to produce images from very sparse point clouds. Here is my series of steps:
I try to circumvent the above error by explicitly loading the corsenet model with batchnorm set to 'set' and then doing the following:
Running with these weights gives bogus results (uniformly grey images as shown below). I wonder how the weights have shifted so much in just a few iterations starting from pre-trained weights which give great results.
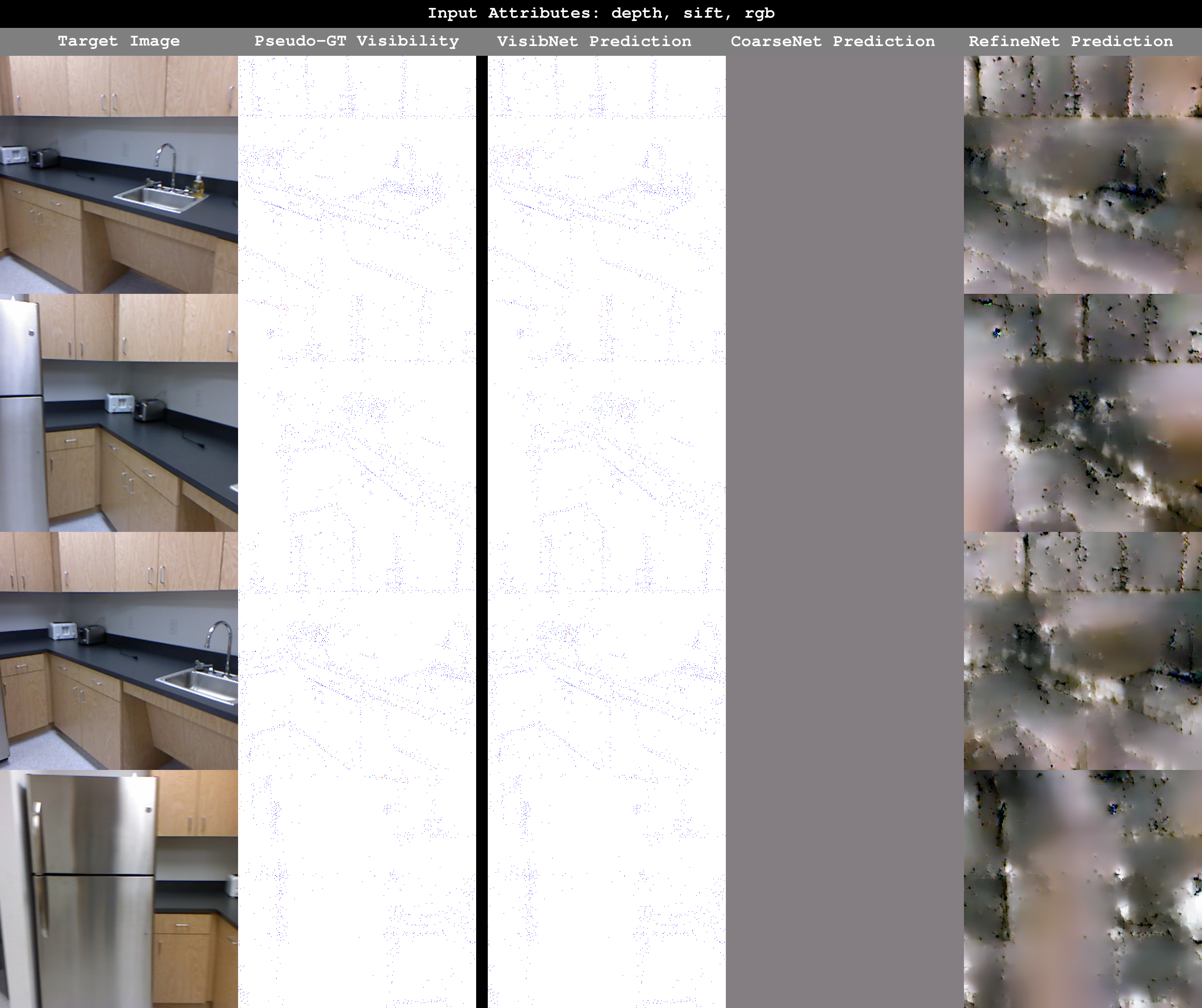
I tried with the example annotation file as well and without the sparsity constraints but it led to similar results. So basically, if I sart from pretrained weights, the model seems to just degrade very quickly even using the same training data. think I might be doing something wrong as these results are strange. Has anyone been successful in training over additional data starting from pre-trained weights ?
The text was updated successfully, but these errors were encountered: